INFO70278
Data Science Foundations |
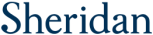 |
|
|
|
Sheridan College resides on land that has been, and still is, the traditional territory of several Indigenous nations, including the Anishinaabe, the Haudenosaunee Confederacy, the Wendat, and the Mississaugas of the Credit First Nation. We recognize this territory is covered by the Dish with One Spoon treaty and the Two Row Wampum treaty, which emphasize the importance of joint stewardship, peace, and respectful relationships.
As an institution of higher learning Sheridan embraces the critical role that education must play in facilitating real transformational change. We continue our collective efforts to recognize Canada's colonial history and to take steps to meaningful Truth and Reconciliation.
|
Section I: Administrative Information
|
|
Total hours: 15.0
Credit Value: 1.0
Credit Value Notes: N/A
Effective: Spring/Summer 2022
Prerequisites: N/A
Corequisites: N/A
Equivalents: N/A
Pre/Co/Equiv Notes: N/A |
Program(s):
Data Analyst
Program Coordinator(s):
N/A
Course Leader or Contact: N/A
Version: 20220509_00
Status: Approved (APPR)
Section I Notes:
Access to course materials and assignments will be available on Sheridan's Learning and Teaching Environment (SLATE). Students will need reliable access to a computer and the internet.
|
|
|
Section II: Course Details
|
Detailed Description
This course offers the opportunity to engage in class challenges, apply coding concepts, and experience hands-on learning as students work through real examples. Students explore the basic aspects of probability and statistics, and learn to program in the open software programming language, R. Students apply a combination of statistics and data visualization into their first regression and analyze its impact.
Program Context
|
Data Analyst |
Program Coordinator(s):
N/A |
This is an introductory course in the Data Analyst micro-credential that forms a foundational knowledgebase for subsequent courses.
|
|
Course Critical Performance and Learning Outcomes
|
Critical Performance: |
| By the end of this course, students will have demonstrated the ability to create and perform statistical mathematical calculations, practice using R programming language, and understand data science career options.
|
|
Learning Outcomes:
To achieve the critical performance, students will have demonstrated the ability to:
|
|
- Explain the difference between probability and statistics in data science.
- Summarize the statistical enquiry cycle and define each stage.
- Explain and apply the concepts and steps in performing a regression.
- Apply and interpret R for basic calculations in data science.
- Describe data science career specializations.
|
Evaluation Plan
Students demonstrate their learning in the following ways:
| Evaluation Plan: ONLINE
| Final Quiz | 100.0% | | Total | 100.0% |
Evaluation Notes and Academic Missed Work Procedure: Grade of 60% is required to pass the quiz.
All projects and exercises must be submitted on or before the date and time specified in
the project brief. Late assignments will receive a 10% grade reduction per day.
If there is a valid reason for the late project, the student must notify the professor no later than the date the project is due to indicate it will be late. If the reason is deemed to be valid, there will be no late penalty.
Work more than one week late (on the 8th day after the due date) will not be graded unless a prior arrangement has been made with the instructor. There will be no resubmission of work unless, under exceptional circumstances, this has been agreed to or suggested by the instructor.
|
Provincial Context
The course meets the following Ministry of Colleges and Universities requirements:
|
Essential Employability
Skills
Essential Employability Skills emphasized in the course:
- Communication Skills - Respond to written, spoken, or visual messages in a manner that ensures effective communication.
- Critical Thinking & Problem Solving Skills - Use a variety of thinking skills to anticipate and solve problems.
- Information Management Skills - Analyze, evaluate, and apply relevant information from a variety of sources.
- Information Management - Locate, select, organize and document information using appropriate technology and information systems.
- Personal Skills - Manage the use of time and other resources to complete projects.
- Personal Skills - Take responsibility for one's own actions, decisions, and consequences.
Prior Learning Assessment and Recognition
PLAR Contact (if course is PLAR-eligible) - Office of the Registrar
Students may apply to receive credit by demonstrating achievement of the course learning outcomes through previous relevant work/life experience, service, self-study and training on the job. This course is eligible for challenge through the following method(s):
- Challenge Exam
Notes: Both a challenge exam and portfolio are required. - Portfolio
Notes: Both a challenge exam and portfolio are required.
|
|
|
|
Section III: Topical Outline
|
Some details of this outline may change as a result of circumstances such as weather cancellations, College and student activities, and class timetabling.
Instruction Mode: Online
Professor: N/A
Resource(s): Course material costs can be found through the Sheridan Bookstore | Type | Description | Optional | Other | No textbook required. |
Applicable student group(s): FCAPS
Course Details: Module 1: Introduction to Data Science - The Data Science Process
- Data Science as a Career
- Career Quiz
Module 2: Probability and Statistics - Overview of probability and distributions
- Overview of binomial and normal distributions
- Examination of the statistical enquiry cycle
- Variability measures
Module 3: Programming in R - Launch and get rolling with R
- Basic stat computations in R
- Working example using the US Arrests dataset
Module 4: Introduction to Regressions - Regression review
- Identifying the response variable
- Creating and interpreting plots
- Creating and evaluating a regression
Final Quiz 100%
|
It is recommended that students read the following policies in relation to course outlines:
- Academic Integrity
- Copyright
- Intellectual Property
- Respectful Behaviour
- Accessible Learning
All Sheridan policies can be viewed on the Sheridan policy website.
In alignment with Sheridan's Academic Integrity Policy, students should consult with their professors and/or refer to evaluation instructions regarding the appropriate use, or prohibition, of generative Artificial Intelligence (AI) tools for coursework. Turnitin AI detection software may be used by faculty members to screen assignment submissions or exams for unauthorized use of artificial intelligence.
The information contained in this Course Outline including but not limited to faculty and program information and course description is subject to change without notice. Nothing in this Course Outline should be viewed as a representation, offer and/or warranty. Students are responsible for reading the Important Notice and Disclaimer which applies to Programs and Courses.
|
[
Printable Version ]
|
Copyright © Sheridan College. All rights reserved. |